Intention to Treat Reporting Concepts
This article discusses the Intention to treat reporting concepts and how it applies to trials. We also discuss the impossibility of obtaining outcome data on all participants and the need to make assumptions about missing outcome values. Finally, we discuss the importance of defining the modified intention to treat (mITT) population and its limitations. Hopefully, this article has helped you make a more informed decision about how to report clinical trial outcomes.
Intention to treat reporting concepts is a critical component of clinical practice. Several articles have examined the importance of reporting the treatment intention of a patient. The New England Journal of Medicine article by Hernan MA, Robins JM, Higgins JPT, and Altman DG summarizes these concepts. However, it should be noted that there is still some disagreement regarding the best practice in reporting outcomes. Nevertheless, the authors are hopeful that this article will clarify the concepts involved in reporting outcomes.
Variability
Intention to treat (ITT) is the gold standard for statistical reporting. This method accounts for the fact that some participants may not receive the allocated intervention, and all outcome outcomes should be reported for the entire study group. Using per-protocol analysis can sometimes result in differences in outcome reports, and grouping participants by treatment introduces bias. While ITT is the gold standard, it is not without its challenges.
Impossibility of measuring outcome data on all participants
This is a fundamental flaw in the way we measure outcomes in clinical trials. Specifically, it is impossible to report data on all participants because of selection bias. This problem was first noted by Bell ML and Robins JM in their 2010 article in the New England Journal of Medicine. The authors further noted that they were unable to report the number of participants who were unblinded.

It is possible to measure outcomes on all participants in an experiment, but obtaining complete outcomes data for all subjects is difficult. The naive ‘per-protocol’ approach limits the analysis to participants who received the intended intervention. This approach introduces bias but is avoided by blinding outcome assessors. It also requires careful analysis of missing data. It is recommended to use the modified intention-to-treat approach when reporting the results of a study in which some participants have been excluded due to missing outcome data.
Modified intention-to-treat (mITT) analysis
Intention to treat is a key approach in the analysis of randomized controlled trials, where patients are included in the study irrespective of whether they were enrolled or not. The exclusion of patients from the study increases the possibility of bias and may lead to misleading results. However, the concept of modified intention-to-treat (mITT) has become widespread in recent trials, and its definition is unclear.
It is important to note that the use of mITT analysis does not necessarily imply the absence of heterogeneity. Some trials do not report data on patients who are not able to participate in a study because of insufficient or inadequate resources. Consequently, the reported response rates of these studies are often too high. In reality, the number of patients who are not able to receive treatment is significantly lower than the rate of responses in trials that use the conventional intention-to-treat approach.
ITT and mITT are complementary methods used to analyze the effect of assigned treatment strategies in trials. Per protocol (PP) includes only the randomized patients and excludes the patients who switched allocation arms or violated the protocol. PP analysis captures patients’ true exposure to treatment. Hence, it is often recommended for trials that report results using a PP approach.
Modified intent-to-treat analysis has several advantages over traditional ITT analysis. For one, it reflects daily clinical practice, while maintaining the balancing of risk factors between study arms. It preserves the study’s power by including participants who were excluded from the PP population. On the other hand, it allows systematic reviewers to extract appropriate data from trial reports.
A study published in JAMA on CD19 lymphoma identified 74 CART trials and reported data on the number of patients not receiving CART after enrollment. The results of mITT and iTT analyses revealed an ORR of 78.3% (95 percent CI: 67.0-89.0%), compared with a 59.0-80% iTT ORR.
Antibiotic randomized control trials (RCTs) routinely use mITT and ITT populations, though PP is rarely used. There are few non-inferiority trials involving antiretrovirals. However, most RCTs of these drugs used mITT or ITT and did not describe their methods for handling missing data. Only 15 studies included non-inferiority RCTs.
The impossibility of measuring outcome data on all participants
A new study has challenged the conventional wisdom on measuring outcome data for randomized controlled trials by finding that outcome data cannot be obtained for all participants. This article, written by researchers from the New England Journal of Medicine (BMJ) and the University of Oxford, describes the problems with this approach. Authors Hollis MA, Robins JM, Higgins JPT, and Altman DG note that this issue is a major concern for researchers conducting clinical trials.
Although the ITT principle states that outcome data must be collected on all participants, this principle is difficult to implement in practice. It can only be achieved by making assumptions about outcome values that are missing for some participants. The result is that analyses described as ITT may not include missing outcome data on all participants. Therefore, such analyses may have bias. In order to avoid bias from missing outcome data, appropriate analyses should be conducted.
The limitations of this approach include the fact that some participants may be excluded from the analysis for reasons other than missing outcome data. Another potential bias arises from a naive ‘per-protocol’ analysis, whereby participants are only included in the analysis if they received the intended intervention. This problem is addressed in the domain of bias arising from deviations from intended interventions.
One of the key challenges of intent-to-treat trials is determining which outcome measures to use. Intent-to-treat studies should use outcome measures that are appropriate for the study. For example, if a portable blood glucose machine does not reliably measure blood glucose below 3.1mmol, this measurement method may not detect the difference between treatments and could misrepresent the true incidence of severe hypoglycaemia.
Importance of making assumptions about missing outcome values
In research, missing data should be handled carefully. In most cases, it is best to assume that data missing at random is of a low probability. If not, you should make assumptions about missing values in a manner that preserves the validity of the results. You should also perform sensitivity analyses to explore the possible effects of varying assumptions. You should also address the potential impact of missing outcomes in the discussion section of your report.
During a clinical trial, for example, you may observe some participants with an improved condition, some with a worse condition, or some participants who drop out before the final outcome. In these cases, you should make assumptions that the outcomes of those who drop out are similar to those of those who stay. By making assumptions about the likelihood of missing data, you can eliminate those dropouts from your analysis without bias.
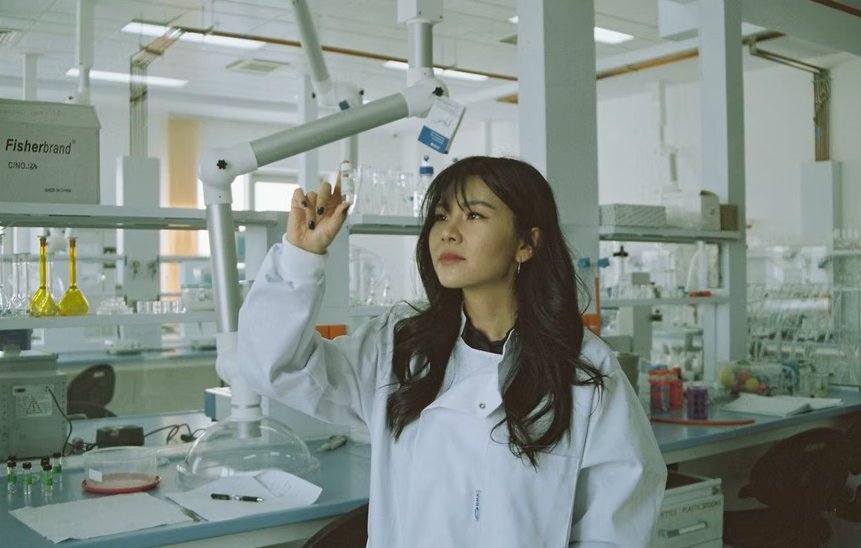
Using a single, ‘per-protocol’ analysis method can lead to bias because participants may be excluded from analysis for reasons other than missing outcome values. The ‘per-protocol’ approach is used in studies where patients are randomized to the intended intervention, but some of these participants may not have completed the intervention. The ‘Bias due to deviations from intended interventions’ domain addresses the potential bias introduced by this approach.
In addition, a study may have to make assumptions about missing outcome values if it uses the modified intention to treat reporting concept. While the ITT principle requires measurement of outcomes across all participants, the process is difficult to implement and requires assumptions about missing outcome values. For example, an analysis that is labeled ‘mITT’ may exclude participants with missing outcomes because the participants failed to return for follow-up. This leads to bias in the data, as the measured and true values of outcome variables are likely to be different.
Intention to treat trials is important because they allow for a greater number of outcomes and a better understanding of their effects. However, this is only possible if participants are all measured, and the proportion of missing outcome data is low enough to exclude bias. Further, sensitivity analyses must also confirm that plausible values of missing outcome values cannot affect the intervention’s effect. As a result, missing data may lead to bias and should be avoided.
Researchers have used the intention-to-treat method in many studies. This method is often used in randomized controlled trials and comparative effectiveness research. However, this approach can lead to errors. Therefore, it is critical to understand the implications of the use of the intention-to-treat method. However, this isn’t a problem if the results are reported correctly.
Researchers have argued for the value of using a shared-parameter model for this purpose. The shared-parameter model assumes that a subject-specific latent variable determines the outcome measurement and the probability of missingness. For example, if a patient has experienced angina, a latent variable could represent the true level of angina. The patient’s perception of pain is not consistent over time, and it would affect the missingness process.
The importance of making assumptions about missing outcome values in the intention-to-treat reporting concept is critical for understanding the effect of a treatment. The observed outcome is the average outcome of the treatment group minus the average outcome of the comparison treatment. The differences between the two groups are then interpreted to be due to the treatment effect. Using intention-to-treat methods can help researchers interpret the difference in outcomes between groups and make the most of the data available.
Importance of minimizing missing responses
Missing data are of three types: completely at random, partially at random, and not at all. The nature of missingness depends on the type of data and the circumstances surrounding the data. For example, incomplete datasets are often representative of the entire population, but their prevalence may differ from observed data. The data that are reported may be biased by a missing response, or it may be a product of an unobservable process.
In reporting concepts, it is important to minimize missing responses. In surveys, strategic nonresponse is one of the most common issues, and this is especially problematic for data that are collected using a survey. Secondary data with missing responses may face similar concerns. As such, researchers should explore the reasons for missing data. While surveys are a prime area for strategic nonresponse, researchers should also take into account the reasons behind such cases.
Intention to Treat Reporting Conclusion
An intention to treat (ITT) report summarizes the results of a clinical trial. It is a critical part of the medical record and must be accurately reported.
Intention-to-treat analysis is a statistical method used to compare the outcomes of experimental interventions. The data is reported with the intention of identifying the treatment that is best for the patient in question. The data in an ITT report include both the treatment group and the control group. If the patients in the two groups switch groups, the results of the study could be skewed.
Intention-to-treat studies are often described using a modified version of the intent-to-treat approach. This terminology may be used when an investigator is having difficulty managing missing data or deviations from protocol. If this is the case, intention-to-treat reporting should be based on explicit statements of post-randomisation exclusions. The authors of these studies should avoid using ambiguous terminology that could lead to misleading conclusions.
